When Kenneth Berger joined Slack in June 2014, the company was at the beginning of its much-buzzed-about ascent. As its first product manager, he managed the product's functionality as it grew from 100,000 to 1M+ daily users — all within his first year. The Twittersphere’s love affair with Slack was booming, too, yielding the kind of 140-character praise companies can only dream about.
So you’d think with all indicators pointing up and to the right, Berger had a pretty easy gig. Not necessarily. Sure, it’s great to be in a place where your growth numbers consistently look good and your users are happy. But with a rapidly accelerating firehose of data, Berger needed to catch and apply meaningful feedback as it flew by. He also learned that no company can rest on its laurels, and that managing his product’s growth might just depend on going out and getting the data he didn’t already have.
In this exclusive interview, Berger shares what he’s learned about prioritizing feedback at Slack, and as a startup cofounder, product manager, and designer before that. He explains why he values goals over metrics, and why founders need to operate like scientists. Most importantly, he shares his tips for sifting through massive amounts of qualitative and quantitative data — from site stats to social media, retention metrics to customer support notes — to create a signal in the noise.
Pursue Goals, Not Numbers
“The problem is not that startups lack feedback, it's that they don't know what do with it, or what they should react to,” says Berger. “More than anything, people respond to what they have history with, or what's in front of them, or what's most easily accessed.” As a result, certain channels are prioritized, while others may be given short shrift, and it seldom has anything to do with what’s actually useful or valuable.
In Berger’s experience, the best way to avoid this pitfall is to focus narrowly and doggedly on your company’s top-line goals. “A lot of people get hung up on the one metric thing. That we all have to be aligned around one certain number that will indicate success. Metrics are obviously incredibly important, but to me they’re just the piece of a goal that makes it measurable.”
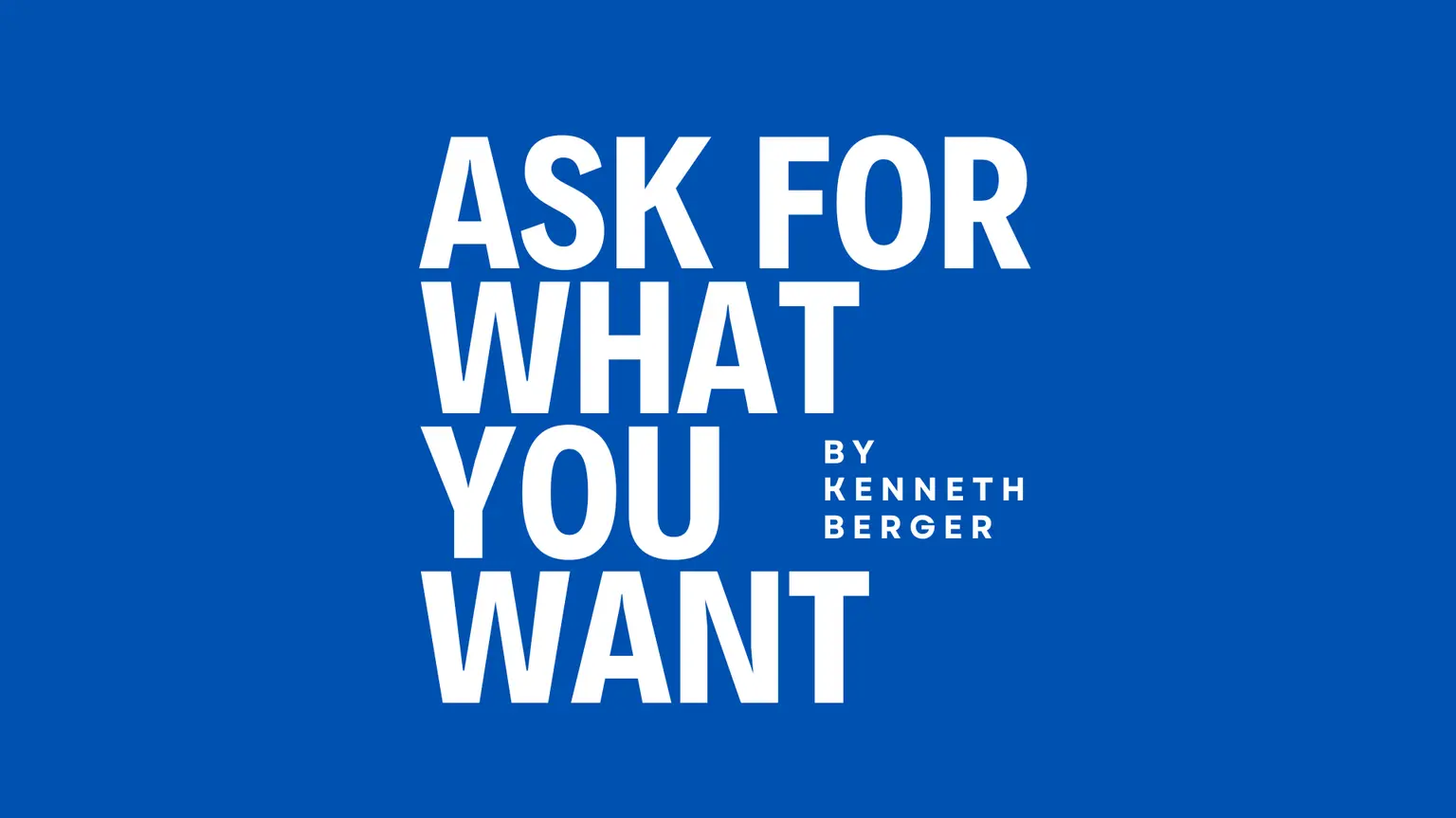
If you like Kenneth Berger's advice, consider taking his How to Ask for What You Want Effectively in Work and Life course on Maven
Berger has spent a lot of time at startups, first co-founding his own, YesGraph, and then joining Slack — and he knows that conditions and minds change incredibly quickly. If you aren’t constantly reevaluating your most important goals for the organization, you may find yourself focusing on a metric that’s no longer particularly relevant or telling.
“That becomes even more complicated if you’re growing your team at the same time, because it's not just that the company's goals are changing, it's that responsibilities are changing. There's a new department, things are getting split up in a different way, and the focus of the company is shifting over time,” he says.
When defining the big goals that should govern your activity, start with this one simple idea: Everyone needs to know why they're doing what they are doing. What goals stem from making sure this is the case? “That’s what the whole company should be worrying about — being able to articulate those things,” says Berger. “Then let each manager or each department figure out what that means for them and what their own metrics should look like."
That’s when metrics become powerful — when the people closest to the work define them for themselves.
"Quantitative focus doesn’t work when people are fixated on numbers someone else told them to pay attention to. Only when metrics are defined by teams and individuals can people push them forward with their own unique perspectives and expertise.”
No doubt, there are a couple of key metrics that will be crucial to nearly any business — namely, retention and revenue. But what those metrics look like, and which other key numbers you’ll track alongside them, vary widely by company. “It depends on the business model, it depends on whether you’re a consumer or enterprise product, it depends on what team you’re a part of within the organization,” says Berger. “I push back against the ‘one metric to rule them all’ view of the world, because if you’re looking at just top-line growth, then how does, say, the customer support team contribute to that? Customer support teams are often incredibly important, so to not give them a metric that’s meaningful directly to them underplays their value within the company.”
Get in a rhythm of regularly revisiting your goals — be it for the month, the quarter, or whatever time frame makes sense for your business. Are you focused on new users? On building value for existing users? Where is the emphasis for the company right now? Armed with that context, you’ll be better equipped to process all of the data coming at you, and to determine which feedback is most applicable at the moment.
Be vigilant, too, about sharing those priorities internally. Inevitably, you'll have to choose one product feature request over another, or move in a direction that addresses just one department’s needs. “Being transparent about all the different sources of feedback and about how you're prioritizing those — or at least explaining why the things at the top of the roadmap are at the top of the road map — helps keep everyone feeling like their feedback is being acknowledged,” Berger says.
At startups, you can’t do everything at once. Fight the urge to try by establishing a clear, and clearly communicated, culture of prioritization.
Smarter Hypotheses Yield Smarter Insights
The process of setting and evaluating your company’s goals is a lot like the work of a scientist, constantly making and testing assumptions. “People think science is more quantitative than it is,” Berger says. “When you set up an experiment, you establish a hypothesis, and you set up ways that you want to test that hypothesis. In business, you can think of that as setting goals, and setting metrics for those goals.”
But a single experiment never really looks at the big picture — that is, you might not have made the right hypothesis, or even picked the right metric to measure it. Part of being scientific about your data is staying aware of that big picture, and knowing when to adjust your theory accordingly.
Early on at Slack, for example, Berger experimented with a few popular marketing mechanisms to see if he could drive more signups. “I was just trying to get started and be helpful however I could. I knew there was a set of best practices for different growth techniques that we weren’t using. So we said, ‘Okay, let's start to build out some of these and see what they do.’” First on the list were retention emails to users who’d registered for the service but not become active users. Next, he tried an experiment with free trials.
“As we built those out and tested them with segments of the audience, we saw improvements, but they were pretty marginal. I think what we understood was that there is a cost to these things. There's a trade-off,” Berger says. And in this case, the trade-off wasn’t worth it. The retention metric may have pointed toward one conclusion, but viewed alongside the bigger picture it started to look very different. “When we looked at the data, we said it wasn't worth the complexity and support cost to generate that additional retention.”
Other times, testing hypotheses has led to critical shifts in Slack’s strategy or messaging. From the beginning, for example, the company made a decision not to talk about the product as a chat app, focusing instead on their broader vision: transforming work, building better communication, and creating greater transparency.
But when they finally did some usability testing, they discovered something interesting. Early on, thanks to a stellar PR push, most people were coming to the site from articles about Slack; they had just read about what the company did, so it was okay for the website to speak in abstract terms. But this didn't work for uninitiated visitors. “People were a bit confused about whether the focus was file sharing or communication or productivity. They needed some clarity, but at that point we didn't even have a screenshot of the product on the homepage. We weren't talking about messaging as the primary integrative framework for the software,” Berger says.
Since then, Slack added a screenshot that went a long way toward educating prospective users. Now that the company and product are a part of the tech zeitgeist, they’ve removed the image from the homepage but explicitly put the messaging functionality front and center.
The fix in this case was clear: Highlight messaging and use that as a bridge to talk about those more ambitious benefits. “It wasn’t that the initial message about transforming how you work was wrong or unimportant. It’s incredibly important. But we learned that you need to tell a story with an arc that makes sense to them."
You need to bring people along on a journey and take them from ‘What is this thing? I just clicked a random link’ to really believing in the mission of the company.
Another hypothesis was how Slack should prove its utility for users. The team started testing a particular strategy — asking prospective clients to go one whole day without using any email, and substituting Slack for all messaging and communications needs. This was in some ways a gamble, asking people to abandon routine while also setting the product up to carry a hefty load. But the experiment was a success. By getting people to break from email for just 24 hours, the Slack team was able to demonstrate definitively how powerful the tool was, giving it strong proof points that it could boost productivity.
Know Your Biases to Keep Them in Check
Another thing we can all learn from scientists? Biases are unavoidable (no matter how open our minds are, we have them), and they'll inevitably color how you interpret your data. Be aware of that, and explicit about how you’ll control the most common sources of bias:
Selection Bias
This is the one that Berger encounters most often in gathering and reviewing product feedback. “It's important to understand, for example, that your support requests are coming only from your most engaged users. If you’re talking to people that are already engaged with the product by definition, you’re excluding all of your potential customers who aren't engaged with the product.”
Or perhaps you’re planning to do some usability testing? Recruiting testers on Craigslist is a logical next step — just keep in mind that the people responding to those postings will not represent the broadest cross section of potential users. “That's not necessarily a bad thing, as long as you understand that it's a constraint. But it also means — and I've been doing that sort of testing for years — you get people who are professional testers. So you learn to recognize them and say, ‘Okay, that’s a loss.’ You need to know that you’re going to get a certain amount of noise in your data, and move on.”
Confirmation or Observer Bias
It’s also perfectly natural to see what you want to see — or the conclusion you predicted — in your data. “Again, no one is to blame for that. It's just a matter of trying to work against it. Think about the alternate hypotheses that data could support. It could mean what you think, or it could mean X, Y, or Z. Proactively push against jumping to see what you want to.”
In fact, Berger sees this process as a particularly exciting part of working for a young company. Employees who’ve come from larger, more established organizations might be used to leveraging data for minor course correction — A/B testing which word on the home page moves the needle, for example.
“Especially if you're in the early stages, you're likely to go on a much more circuitous journey with your data. Be willing to go on that journey, and start it as soon as you can. It can teach you new things about your product or your customers.”
This data-based journey played out in particularly dramatic fashion for YesGraph, the startup Berger co-founded prior to joining Slack. The product was originally designed as a tool to help recruiters source candidates from their social networks, but he found that users weren’t making it through the adoption process — and the company just wasn’t scaling.
“We actually had a really interesting product around finding the right people to invite to your social service. We had so much data that we were using to understand people and who might be most receptive to connecting into the service. Turns out that's something very valuable for a bunch of social services,” Berger says. The company has since gone in that new direction, and thrived.
Reporting Bias
While observer bias is all about seeing what you want to see in your data, reporting bias means only looking at the types of data you’re most comfortable with. Often, this manifests as deemphasizing data sources that are a little harder to see, more complex to dive into or less quantified. “This comes up a lot when making trade-offs for different product decisions. You might have one source that's very quantitative, so you're focused on measuring that — and that source speaks to the upside. But there may be a lot of qualitative measures that reveal the downside. Your product may be driving growth, for example, but how is customer satisfaction? How is support volume?” Berger says.
You’ll always face the challenge of weighing data that’s coming from very different places, but it’s worth it to look at your product decisions from multiple angles. “Being clear-eyed about the pros and the potential cons of any product decision, and understanding that data is going to come from a lot of different places, really helps you keep a clear view,” he says. “I think it helped us make a lot of smart decisions at Slack, around those engagement emails that we experimented with for example. We could still be managing all that complexity today, but it just wasn't worth it when we looked at the big picture.”
Combining Qualitative and Quantitative Data to Make Them Both Stronger
Still, as you build your hypothesis and begin designing your test, you’ll need to start with the data sources you believe will be most instructive. “It’s about what questions you have, what opportunities you have to collect feedback, and what will be the right way to do that,” Berger says. When it comes to looking at quantitative vs. qualitative feedback, he operates with a general rule of thumb:
Quantitative data can tell you if something is wrong, and qualitative can tell you why.
While you’re prioritizing a handful of data sources, should you still be tracking the rest? Otherwise put, in a world where everything can be instrumented, is there an argument for capturing everything, just in case?
“It's a question of noise. Often, capturing data is not free. There may be an explicit cost, or at least an opportunity cost,” Berger says. Even when data does come “free” — adding a new metric to your app’s analytics dashboard, for example, is easy — consider whether it’s worth the distraction. That’s often an invisible cost.
“It's very easy to instrument everything, the same way it's easy to look at every piece of feedback on Twitter. You are always going to be able to find some concerning bit of data, be it qualitative or quantitative,” he says. But if you’ve been clear about your goals, and clear about your hypothesis for how to achieve them, you can resist the urge to scramble and change your product because one person doesn’t like a feature. There may be times when real-time Twitter feedback is the source you’re prioritizing — right after a new release, for example. But you need to be discerning about when that kind of data suits what you’re testing.
“You need to understand and be explicit about your data sources. This is the most important thing, and these other things are less important. It doesn't mean you don't pay attention to the other things. If you think your latest feature is important, but it starts blowing up customer support, you need to listen to that. But you don’t have to pay attention to everything equally,” Berger says.
Gather Data You Don’t Have
More often than not, when your quantitative data surfaces an issue with your product, you’ll find it with a quick glance at your analytics dashboard. “Big problems are obvious. They’re not subtle things,” Berger says. Qualitative feedback, in many ways, is trickier. Remember selection bias? Unlike site traffic numbers or retention data, you’re inherently seeing just a piece of the big picture when you look at your support feedback or social media feeds. Getting actionable qualitative data is often a matter of going out and finding it proactively.
When Berger joined Slack, he faced what seems like an enviable problem: Product feedback was great. “Early on, I sort of said to myself, ‘Okay, if everyone on Twitter says this is the greatest thing since sliced bread, what am I here for? How am I supposed to add value?’"
So he sought out the people who weren’t saying anything. He knew that the company’s next step needed to be supporting larger teams. At the time, Slack users topped out at teams of 300 or 400 people. So Berger went to visit the largest teams he could find. “It was incredibly illuminating, because their experience was just so different than those very engaged users on Twitter who — surprise, surprise — were very similar to our team itself,” he says.
What he learned was that, while these teams were getting a lot of value out of the product, they also had consistent frustrations. And most of those were actually pretty quick fixes. “It would have been so easy to just keep looking at the single source of feedback, which was so nice — everyone loved Slack! But that wouldn’t have been good long-term.”
You always have to move on to the next thing, and the next thing is always tackling that next stage of growth.
While gathering this kind of forward-looking, highly qualitative data may seem like a radical departure from the clear-cut comfort of your analytics dashboard, it’s really not. Here, too, you should start with a hypothesis and then determine the metrics and process you’ll use to test it.
“My hypothesis was that we weren’t serving those larger teams as well as we could,” Berger says. He quickly identified Slack’s largest accounts, starting with the low-hanging fruit in the Bay Area, and reached out. “Within a week or two, I was out there with a set of questions that really were not focused around any particular problem, but just around understanding how those teams used the product.”
The questions themselves were straightforward: How do you use Slack? Who uses it within the organization? What do they use it for? What other tools do you use, and how does Slack fit into that mix of different tools? How did you adopt it?
For example, When Berger and the team first started conducting these interviews, the product was built with a certain number of default notifications, as well as a way to toggle notification preferences. So if you were working on team, you’d receive a certain number of emails or desktop notifications as your colleagues took various actions. This worked brilliantly for small and new teams, where people wanted a lot of notifications to keep tabs on everything going on. But the same set of notifications turned out to be overwhelming for large teams.
This was feedback Berger heard again and again, and as a result, Slack created a different set of default notifications for larger teams. This scaled down the number of pings people received throughout the day while still giving them a handle on their personal messages and their team’s overarching activity. This made a big difference for companies of greater scale.
While quantitative data can be easily presented in a dashboard format, how do you digest qualitative feedback like “I’m getting too many notifications,” and share it with a team?
“The easiest thing I can say is that your biggest conclusion will be obvious,” Berger says. “A lot of people struggle with how to use qualitative data, because let's say you go out and do interviews with five people. You say, ‘Well that's only five people. How do I know what to pay attention to, or whether this is important?’ That's where all that preparation upfront becomes so important. You didn't just go to five random people. You thought about what your hypothesis was, and who the right people would be to go to and test that hypothesis.”
Sure, those five interviews might not yield unassailable insights about every type of user. But if, like Berger, you go to large teams, you can only make a conclusion about large teams. If you speak only with highly engaged users, you’ve gathered meaningful data about engaged users.
Furthermore, there will be times you encounter user feedback that doesn’t need to be tested or explored with a survey. It just makes immediate sense. “Sometimes you'll see one person using your product in a way that's just a crazy, out-there use case. But other times you'll see someone in a particular use case having a serious problem. And you don't need to see anyone else, because you understand that naturally that's going to happen to everyone,” Berger says.
During his interviews with Slack’s large-group users, he encountered one such problem: The product’s autocomplete feature was jumping the gun on user names. Someone typing a simple word like “don’t” would instead autocomplete a user name like “@dondraper” or “@donjohnson.” Berger didn’t need to gather information from more users to take action on this issue; it was clear that any team of a given size would eventually encounter this glitch. “You see a case like this, you understand it, and you can extrapolate how important it’s going to be to fix it.”
Berger operates with another guiding belief: There are certain things in your product that you want everyone to be able to do.
These will always be use cases so crucial to your product’s success that even a small sample size of users can give you enough data. “You can imagine that everyone should be able to get through onboarding, for example. If you've already decided that you want the trial of Slack, then in theory we want one hundred percent of those people to be able to get through. In that sense, if you take it to five people and only three of them can do it, it's clear that you have a problem,” he says.
Of course, five users won’t always be enough to get you the data you need. “Again, it's more a matter of matching the right tool to the right kind of problem.” Getting out of the office and meeting with users is harder, but it can yield big-picture feedback on what needs to change or where to take your product next. On the other hand, for subtle changes you might want to gather data from a larger group. “If you want to get an incremental bump in your onboarding flow, for example, work off analytics, or try a survey or a questionnaire on your home page.”
Whatever the source, Berger advises earlier-stage startups not to worry too much about whether they’ll know what to do with their data. “Choose your hypothesis carefully, choose who you talk to carefully, and it will almost always be clear what to do next.”