There’s heaps of commonly-held wisdom that, at the beginning of a startup journey, founders should spin up an MVP as quickly as possible to start accumulating feedback. After all, once founders latch onto a startup idea, they’re keen to just start building, especially if they’re very technical.
But for the co-founders at Labelbox, product building was a slow burn. Although original co-founders Manu Sharma, Brian Rieger and Daniel Rasmuson are all extremely technical (thanks to their background in aerospace and software engineering), their energy in the earliest days of Labelbox was spent on deep customer discovery work, rather than being heads-down building an early prototype.
That fierce commitment to the idea validation and customer discovery stage — rather than jumping feet-first into building — allowed the Labelbox team to collect valuable early feedback to fine-tune a product that would immediately shoot the startup to hockey-stick growth.
Now a Series D startup with over $188M in funding, Labelbox has emerged as a leader in the burgeoning industry of data labeling. Its software is used to annotate large batches of data; more specifically, it helps people identify and categorize data. To understand its product, think of a picture of a car. A window pops up and asks if you want to label it as a Tesla versus a Ford. Its technology is then able to label all pictures of Teslas accurately through AI.
“The distinct problem we set out to solve was to create a collaborative labeling system,” Sharma says. “Despite launching in 2018, all of the state-of-the-art tools at the time were all desktop applications. We knew AI was going to get bigger and that data labeling would only become more important, and we wanted to build a solution that would not only manage large amounts of data, but could allow multiple users on one team to log into a cloud-based platform and be able to collaborate on the labeling process.”
Also serving as Labelbox’s CEO, Sharma unspools the history and entrepreneurship that went into building Labelbox, recounting his transition from an aerospace engineer into the world of neural networks, how he leveraged insight from his past job to validate a distinct problem of data labeling, and why he and his co-founders prioritized customer discovery overbuilding in the earliest days — eventually nabbing large corporations like Condé Nast and Allstate as some of its earliest customers.
Let’s rewind the clock.
EXPLORING IDEAS
Labelbox’s story begins nearly a decade ago, back when Sharma and his co-founder were students at Embry Riddle Aeronautical University. The two would eventually go into tech and build AI-powered solutions together, but as an aeronautics and aerospace engineer, Sharma was focused on using AI for an entirely different project.
Artificial intelligence was not the ubiquitous technology that it is today. In the early 2010s, it was largely limited to clunky yet simple models, that focused on neural networks and training computers to read patterns within a set of data. It was through these basic training models that Sharma first familiarized himself with AI.
“At that time, working with neural networks was still archaic. This wasn’t even too long ago, it was around 2009 and 2010, but one of the best ways to work with neural networks was to use MATLAB or Simulink software packages that are widely available at educational institutions,” Sharma says.
“I was very intrigued by these systems because you didn’t have to learn equations to figure them out or train them. You just fed them data and it would start mimicking behaviors it would see from patterns in the data,” he says.
Sharma then spent a year or two testing and building solutions for the aerospace industry with these neural networks, working towards autonomous flight.
His co-founder and current Labelbox COO Brian Rieger had a similar path. The two were both students at the same time and worked on class projects like these together before going their separate ways after college: Sharma took off for product jobs at startups in the Bay Area, and Rieger ended up in a data science role for Boeing in Texas.
“Fast forward to 2017, when I was working at my second startup in the Bay Area, a satellite imaging company Planet. I reached out to Brian, who was still at Boeing. We both had a realization around this time that the next stage for our careers would involve working on interesting problems together,” Sharma says. “We made an agreement with each other that no matter what, we were going to quit our jobs and build a company.”
But the pair didn’t set their sights on a venture-backed business at first, in fact, their intention at the time was to work on something that would amount to a small business. The only question that remained was what idea should they pursue?
“There was a ton of interest at this time around how AI could be used in novel, groundbreaking ways like self-driving cars,” Sharma says “But one of the interesting things that was happening around me at Planet was the intention to build computer vision applications based on the large batches of data we would collect every day from 300-400 daily satellite images. The way our engineering teams would go about building these applications was by exploring what data they should even be using. There was this idea around labeling and it was a very new concept.”
“To train these neural networks on how to correctly label data — such as accurately describing what information can be pulled from a satellite image — humans have to impart intrinsic knowledge about what certain objects look like and how they can be identified.”
Sharma thought back to his self-flying airplane in college and realized that the paradigm hadn’t changed. Neural networks were being trained to understand data in the same ways: feeding data into a model that would become trained to mimic the behavior it was told to do.
“But what did change is the computation and the algorithms used to train these networks became so much more powerful,” Sharma says. “And more importantly, it seemed to be improving faster than Moore’s law.”
This realization was enough to spark the seed idea of a new business to pursue. Sharma started to use his days at Planet soaking up all of the information he could around this problem space, taking notes on how internally the team was exploring ways to go about building an ML infrastructure and scaling AI.
Sharma started to poke around the market to see what alternatives were available as well. There was CrowdFlower, whose technology used human intelligence to do simple tasks such as transcribing text or annotating images to train machine learning algorithms.
“Everything else was basically just open-source tools or desktop applications,” says Sharma. “But lo and behold, none of these things were appropriate for solving Planet’s problems internally. The team was looking for a more robust and comprehensive solution to organizing and labeling their data.”
Hence the origination of the Labelbox idea: “Our biases have always been toward building tools. We’ve always loved building tools and admired great ones. So we asked, ‘What can we do here?’ Maybe we can actually come up with a product that will become an interface for these neural networks,” says Sharma.
Shape the early product off of pain points
By the middle of 2017, Sharma and Rieger remained firm in their conviction that AI was just going to keep growing and there were immense opportunities in the space.
“The bet was that more and more businesses are going to need to train these models, but labeling data is a very human affair. It requires human judgment, and humans are not always going to agree,” he says.
With a loose idea around data labeling and a keen interest in neural networks, Sharma and Rieger set out to fill the pain points caused by the void of collaborative labeling systems for data.
“At the time, there was no collaborative solution I could find where I could invite X number of people, and assign them different roles and permissions that would allow them to work on static data collaboratively,” Sharma says.
Sharma thought back to the pain points he observed the engineers and team leads at Planet struggle with when trying to sort through large batches of data.
“All of the state-of-the-art software at the time was desktop software, where if you wanted to solve this problem of data labeling, you had to install the software on every computer and with a thumb drive, allocate 10, 20, however many images to one person to label. And this was in 2017,” Sharma says.
“It felt intuitive to us that if we made software that was easy to access from anywhere, allowed multiple collaborators to use it at once, and made it scalable for growing businesses, it would solve nearly all of the problems of an internal eng’s team’s work,” he says.
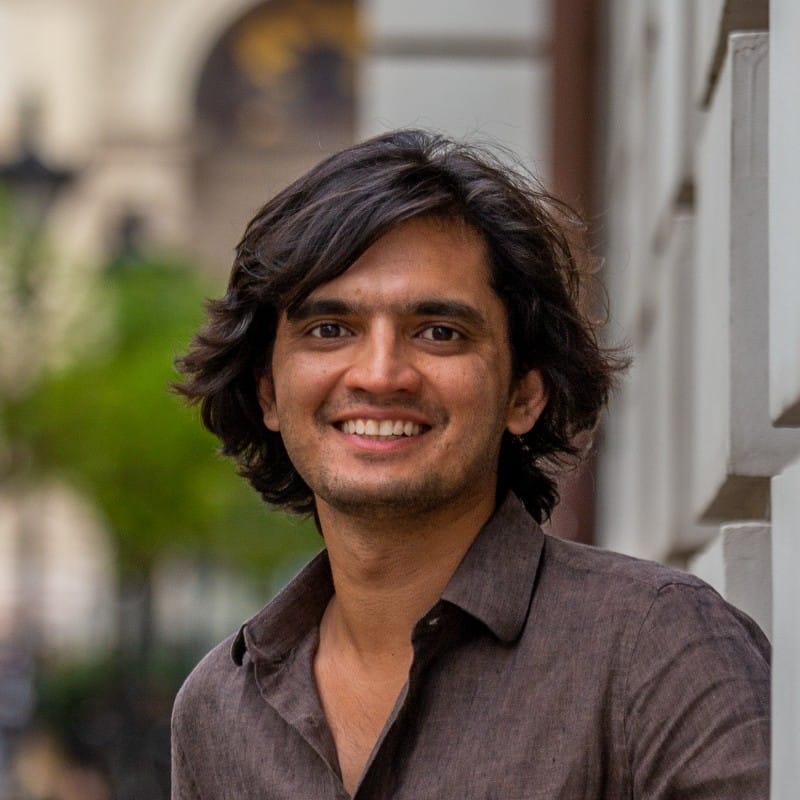
VALIDATING THE IDEA
Even with the headstrong conviction that AI and data labeling were going to become a massive market in the near future, Sharma and Reiger found themselves quite early to the party.
Sharma knew from his current job at Planet that at least one internal team was tinkering with building data labeling infrastructure, but to validate their belief that a collaborative data labeling tool was a product people were willing to pay for, they would need to find others.
Take a pulse check from the experts
To get the ball rolling on an early customer discovery process, Sharma and the co-founding team decided to start pitching their idea to a niche subset of experts who worked with artificial intelligence every day.
Sharma felt it was important to establish their credibility in these meetings with AI experts, so he pulled a page out of his old product management playbook. Leveraging his design skills, he created mock-ups and early sketches of what the Labelbox product could look like and how it would work. “That gave us a little bit more fidelity into a solution space and validated that there was room for us to build.”
With a static sketch of their data labeling solution in their hands, Sharma and his co-founders set out to speak with these experts.
“We started talking to a handful of AI startups in San Francisco or within our network. We would reach out to them with our idea for Labelbox and a mock-up and ask ‘I would love to learn from you how we can solve these problems,’” says Sharma.
They got very mixed feedback from this early cohort of folks.
There were many experts in this field that had tried to solve the problem themselves, and they told us that Labelbox was an absurd idea that was never going to work. They said it was impossible to create a general product solution for such a bespoke problem.
If all the feedback was doom and gloom, Sharma says they probably would not have kept going. But the point of this exercise, according to Sharma, was to get a pulse check on what the AI community cared most about at the time, and how experts were approaching building their own collaborative data labeling tools. And as it turned out, amid a chorus of no’s, there were a few fans.
“Other AI teams would tell us that what we had was really interesting and they would love to use it,” Sharma says. “Almost always, they were looking at it for their own particular use case, whether it was labeling medical images or geospatial drone maps, but still said they’d love to use any general prototype we had.”
Create a framework for collecting feedback
Armed with knowledge about how the top AI experts were approaching building a collaborative data labeling product (or more accurately, how experts were not attempting to build this) the co-founders’ next step was to talk to a slightly wider audience.
“We didn’t have any discrimination on what a good person to talk to looked like or what title they had,” says Sharma. “What we were looking for was a technical leader at any organization where we could get a warm intro, whether that was the CTO, a VP, an ML engineer, or a product manager who was directly building a computer vision application.”
While the co-founders established some criteria of what made someone a good candidate to talk to and eventually pitch, they decided that they would also need a proper framework to measure any feedback they collected.
Sharma shares the two signals that he and his co-founders used as guideposts from early customer conversations to affirm that the Labelbox idea could be scalable:
- Problem validation. “We were in a vacuum of our own experiences in the data labeling world, and we had formed assumptions and hypotheses around the market. Hearing from other people that yes, they were frustrated with the desktop tools available and yes, they were working on building a solution internally and yes, they were planning to do more labeling in the future validated our assumptions on a macro level.”
- Solution validation. “Some teams were open to showing us their internal tools, which was huge. We were able to map out their mental models, how they approached data types, data structure, and the same workflows for data labeling that we were working on. This was an even more powerful signal to us because it gave us the insight that others were also trying to solve the problem we presented.”
Build guardrails into questions
What makes Labelbox’s path to product-market fit stand out is how much time the co-founders dedicated to problem discovery in the earliest days, rather than being heads down building.
“We wanted to spend most of our time aligning on the right problem we were trying to solve and turning around and validating that with future customers so that when the time came, we could just go for it and build,” says Sharma.
In product discovery, asking the right questions is more than half of the work. The key is to not give out solutions to people, but to allow them to discover problems in a more abstract way.
To make sure they were doing this in an artful way, Sharma divulges not just what questions to ask in early validation conversations (spoiler alert: it's all about the open-ended ones) but why founders (especially technical ones) can benefit from them.
- Good questions are open-ended but set guardrails. “These are questions like ‘How are you going about labeling your data?’” You can learn a lot more when you leave the question open-ended, but the key is to set guardrails so the conversation doesn’t turn tangential. In this case, the parameters are data-labeling.”
- Good questions disarm people. Asking yes or no questions can imply judgment that’s not really there. “A question like, ‘Do you think you’re happy about investing your time and money on building labeling infrastructure?’ can put people off,” Sharma says. Instead, lead with empathy. “A better way to ask the same question is ‘We spent a lot of time building labeling tools before and thought there could be a better way. We are wondering if you can share some ideas on challenges you’ve faced?’”
- Bad questions are closed-off: “Not only does this not provide you the insights you can get from an open-ended question, but you’re presenting only two logical sequences that a person can follow. Are you building an internal tool? Yes or no. It closes off the conversation and stunts it from leading to somewhere useful.”
While the meetings added up and the stack of feedback started to pile higher, Sharma says his team needed a watershed moment to push into building mode. That moment came during a business meeting where one of Labelbox’s co-founders, Dan Rasmuson, almost got poached.
“Dan and I went into an in-person event with the CTO of a major medical diagnostic imaging company,” Sharma says. “The CTO told us he actually wanted to hire Dan as a developer to build and improve their internal tool for data labeling. We thought if somebody is willing to pay Dan to improve this internal process for them, that was enough signal for us to get started.”
This was the final piece of validation they needed before setting out and building V1 of the product.
There are two things that I firmly believe about getting past the validation phase: 1. You have to have a wildly crazy idea before it becomes a breakthrough. 2. Your idea has to be both contrarian and right for it to find success.
FINDING EARLY CUSTOMERS
After putting in long hours sketching mock-ups of a non-existent product and hustling to get warm intros, Sharma and his co-founders felt confident that Labelbox was a product that would sell.
“As we quickly threw together a prototype, getting the first version of a working product precisely right wasn’t a huge concern,” Sharma says. “Because we were confidently aligned on our problem statement as a team, and we were convinced that any eventual users would be facing the pain points we were trying to solve.”
Rather than put the resources toward a big official product launch, Sharma and his co-founders created an app where people could sign up for free and try a beta version of Labelbox. “At that time, we just wanted to share what we had built with the ML community as quickly as possible and find out if it was useful or not. We took the quickest and shortest path to reach users,” says Sharma.
Instead of trying to get splashy press coverage, the Labelbox team focused on seeding the product organically.
“We would embed ourselves into these conversations within the community with the assumption that qualified people would discover it or we would brand on Google searches and people looking for a solution would see us,” Sharma says. “Day one of Labelbox was dropping the beta app into different machine learning forums on Reddit.”
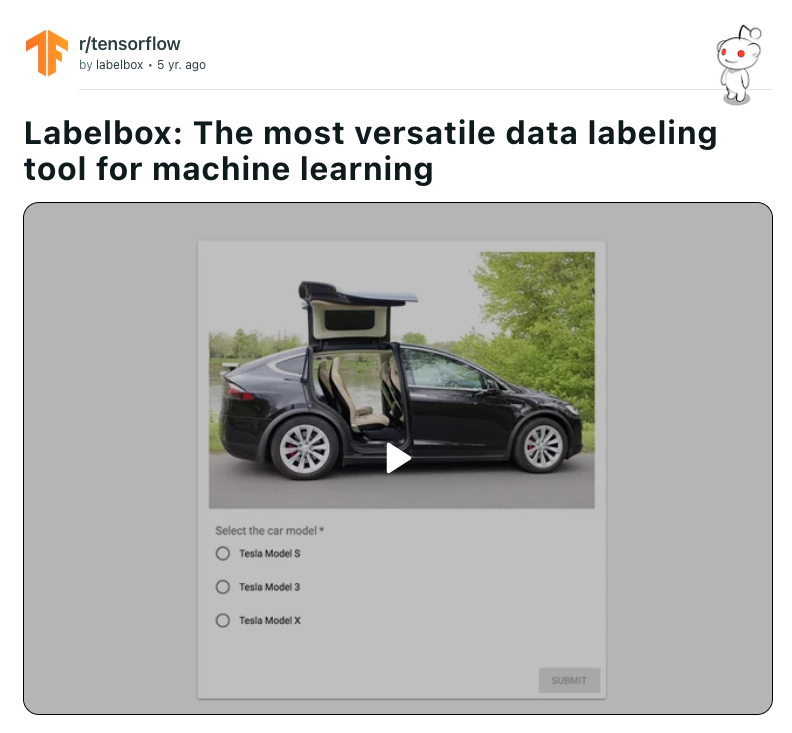
For founders looking to get creative around building awareness and product discovery, Sharma shares a few more of the savvy ways his team was able to bring Labelbox to the customers, not the other way around:
- Question and answer websites. “We would research related questions people had about data labeling on sites like Quora and we’d answer these questions by offering Labelbox as a solution.”
- Wikipedia. “I found a way to put ourselves as one of the bullet points on a Wikipedia article about data annotation tools. We saw a 40% boost in traffic just because people were using that article as a route to find these tools.”
Test pricing from the get-go
Within a matter of days, Labelbox found its first users. For Sharma, the next logical step was to immediately start testing the waters on price. This commercial-oriented mindset proved to be advantageous in bringing in early revenue.
Sharma and the team were strategic about tracking each of the early users they saw using the product, and they leapt at the chance to ask them for feedback. They emailed these early customers to ask questions like:
- “How did you hear about us?”
- “Why are you here?”
- “What would you like us to work on?”
By collecting early feedback, they were able to finetune exactly what features in their product they could put behind a paywall, and gauge just how much interest there would be to pay for something like Labelbox.
“We didn’t have a pre-thought-out pricing system or accepted bids or anything like that, but we knew we needed to charge and start testing pricing,” Sharma says.
The co-founders settled on offering a free version and a pro version of Labelbox. “The pro version included extra stuff, like more data capacity,” Sharma says. “And we started charging $100 a month. As demand increased, we were not shy about raising prices either. In a span of weeks, we were charging $200 a month, and customers kept coming in.
Three months after the unofficial beta launch on Reddit in January, Labelbox started to see daily active users. “We signed up for the free version of Hubspot and started building a pipeline to track customers to convert,” Sharma says. “We had a rough pricing system around the free and paid version. Anyone who wanted pro would talk directly to us co-founders.”
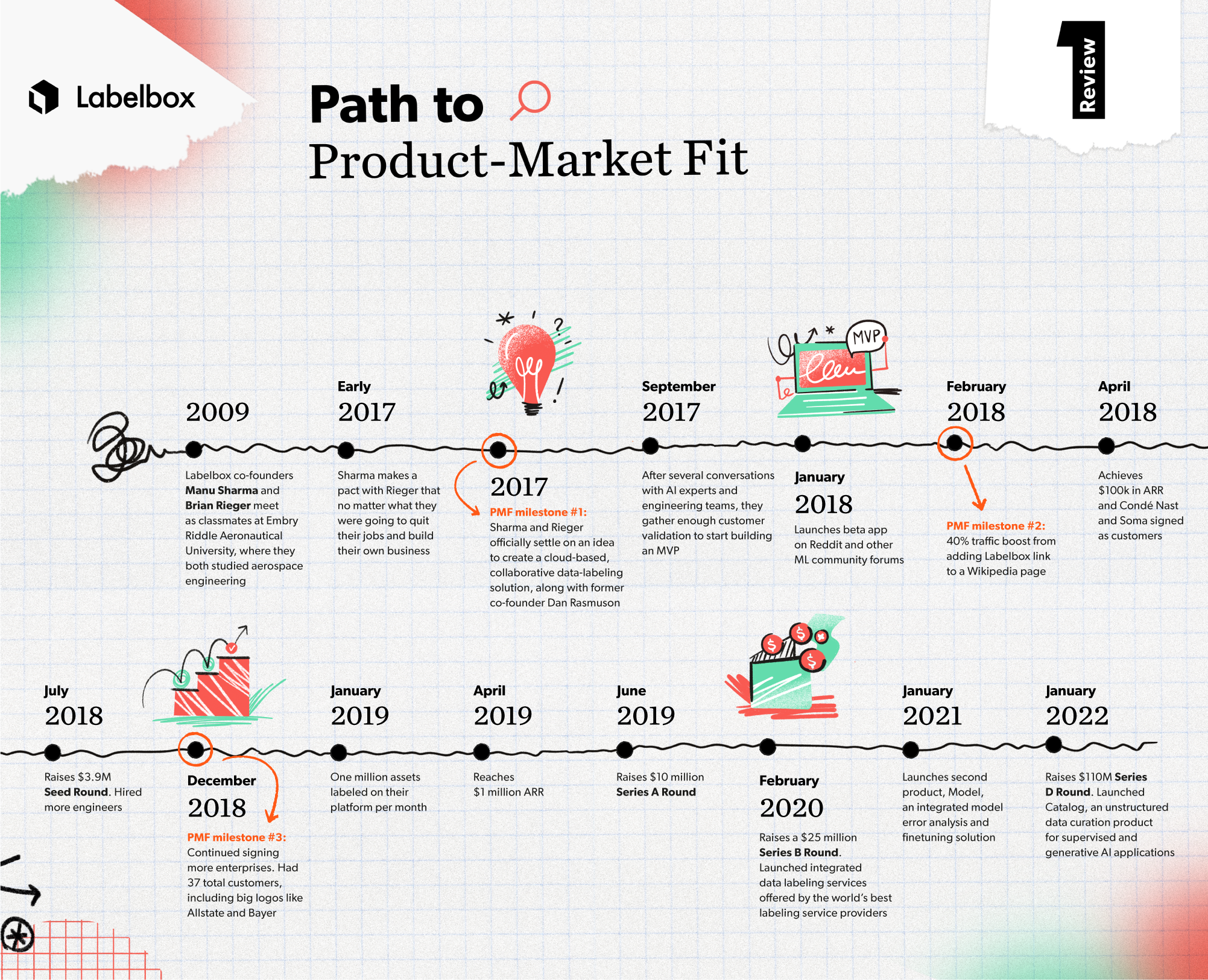
Zeroing in on enterprise
Labelbox had launched with a self-service model in mind oriented towards smaller orgs — but the product was garnering attention from giant firms right off the bat.
Many of the early customers that found Labelbox through Wikipedia links and community chat forums were enterprises. Publishing giant Condé Nast was among the first to reach out to Sharma and his team asking to sign a contract.
“When we saw Condé Nast come inbound in our second month of operating, we went to go speak with their team,” Sharma says. “I remember in a meeting, we signed $20,000 off the bat. Our prices started at $100 a month for our services and in the span of 60 days we were signing a $20k contract a year. We became really confident in our pricing model at that point.”
Qualify and prioritize
With Condé Nast signed, $100,000 in ARR, and more big logos coming through the pipeline — all by April of 2018 — Sharma and the co-founders implemented a simple system to make sure they were organizing their top-of-funnel efficiently.
“We started prioritizing the enterprise logos that were in a pipeline or signup list and sent them emails asking what else we could solve for them,” Sharma says.
This meant that the first four to five customers at Labelbox were enterprise companies.
The founders got into a familiar rhythm. The team would build out new features (mainly at the request of their companies), promote them to their mailing list of existing customers, and follow up with additional outreach to the rest of the inbound requests in the pipeline.
After taking ample time to validate the idea and build V1 of the product, suddenly the velocity kicked into high gear. “We were shipping things real fast. I think Dan shipped half a million lines of code in the span of a year,” Sharma says.
It was a process that was running fairly smoothly, but in order for Labelbox to move at an even greater clip, they needed more engineers. Sharma and his co-founders set out for their first round of fundraising.
In July 2018, Labelbox closed a $3.9 million seed round led by Kleiner Perkins, with participation from First Round Capital. “The seed round was a big milestone for us because we were seeing a lot of community traction. We hadn’t initially planned to raise venture capital, however, raising capital was the only way to keep up with the demand while offering a good customer experience. Within three months of launch, we started getting investor interest and even got an offer to join Y Combinator. We ultimately decided to close the seed round and focus on building the company,” says Sharma.
Sharma wasn’t kidding about the investor traction Labelbox received after the seed round. With fresh capital in the bank to hire and expand the team, Labelbox continued building, iterating on the core product, and signing more enterprises as customers. By the end of 2018, Labelbox had 37 total customers, with contracts from companies like Allstate and Bayer that brought in upwards of six figures.
It wasn’t just customer contracts that continued to grow. By the beginning of 2019, more than one million data assets were being labeled on their platform every month. By the end of the first quarter of that year, Labelbox was on the verge of getting to its first million in annual recurring revenue. “Which meant that within less than a year, we went from just an idea on paper to a million dollar run rate.”
The snowball effect continued. By June 2019, Labelbox raised a $10 million Series A led by Gradient Ventures.
Later that year, investors from Andreessen Horowitz reached out to Sharma and Rieger. “They had been researching in that space and had built a thesis that the market needed a software player that could be a disruptor.” Through many back-and-forth conversations, meetings and exchanges, Labelbox chose the firm to lead their $25 million Series B round.
“The way we approached fundraising was by prioritizing the chemistry we had with the people we met,” Sharma says. “And as long as we were at reasonable valuations and metrics and parameters, we will just do a deal and that’s how we did our Series A and B.”
LOOKING FORWARD
Since Labelbox’s launch in 2018, the data-labeling tool has grown into a Series D startup that has raised over $188 million in funding and handles over a million digital assets uploaded to their platform every month.
The original Labelbox idea has expanded into a multi-product suite. In 2020, Labelbox released a product called Boost, which is a marketplace for data labeling services. And in early 2021, Model was released as a debugging and performance-enhancing tool. Their data and analytics tool, Catalog, was released in 2022. “There were always undercurrents of us trying out these ideas, but it really felt like we had the most product-market across our whole suite in the past few years.
The AI ecosystem continues to grow, and as the company experiments with releasing products with generative AI, Sharma is sure that Labelbox will grow right alongside it.