Here’s a familiar trope in tech. Founder launches a startup. The startup begins building a product. It urgently needs to get that product to market. To do so, the startup needs technical talent — yesterday. Engineers interview at the startup. The hiring manager (or the founder or VPE early on) likes a candidate and makes an offer. The engineer asks for more — substantially more. The hiring manager needs this “critical path” hire and agrees. Rinse, cycle, repeat — and more engineers join. Headcount increases. The product launches. The company grows. Now it’s time to formalize performance levels and compensation packages, but they’re all over the map.
If this sounds familiar, it’s because it happens all the time. Under pressure, startups have a need for speed that makes freestyle negotiations or plug-and-play comp data resources attractive. But it doesn’t have to stay this way — and it can’t if a company wants a sound comp philosophy, one that prizes equity, transparency and employee happiness. Enter Jeremy Stanley, Udi Nir and Guissu Baier, the VPs of Data Science, Engineering and HR, respectively. They’re the multifunctional task force that Instacart assembled to retrofit the company’s compensation practices. Its system wasn’t broken, but they wanted it to be exemplary. So they got to work.
Flash forward a few years. Instacart Engineering, a team of around 90 people, has been using the trio’s compensation system — and has seen promising results. Years ago, there were compensation discrepancies — to the tune of tens of thousands of dollars — between engineers of the same rank. Now, 83% of Instacart’s engineers are within 5% of their target compensation for their defined roles and 95% are within 10% of their target. The trio admits that their efforts are still a work in progress, but their changes have not only improved the candidate experience, but also have helped Instacart calibrate and project how its current team can grow.
In this exclusive interview, Stanley, Nir and Baier deconstruct how they are building a more transparent and trustworthy compensation culture. They start by sharing when and how they use third party comp data as an input to create a customized algorithm. They also break down the different sources of data to add in. Lastly, they share how to integrate this information into their hiring process when salary and equity is discussed — and how to stay competitive as your company and market change. Let’s get started.
Instituting a Data-Driven Approach to Compensation
Before Stanley, Nir and Baier began their effort, Instacart used data to determine compensation, but it wasn’t as confident in its methodology. At its stage of growth, the company found it needed a more sophisticated approach. “There will be different signals that you need a better methodology for comp. For us, we noticed that conversations with candidates generated a lot of questions and back-and-forth. If we couldn’t explain our methodology cleanly, there was doubt. That can quickly turn to distrust,” says Baier. “While our early methodology had flaws, we weren’t far off the mark. We just knew we wanted to be more consistent about compensation — and make it clear that we operate on a methodology that is clear and competitive.”
“So we started by holding ourselves to the same standard on decision making with data with respect to comp. We felt we owed it to our employees to be able to explain clearly how we arrived at the numbers — not just for any tech company, but for Instacart specifically,” says Nir. “That meant a new process to hit that clarity and consistency. That can be painful in the short term, but if you don’t do it, it’s excruciating in the long term. Because when you grow, that pain increases exponentially.”
Now, before a candidate meets a hiring manager at Instacart, the company has three types of information at its fingertips: market data, interview data from past candidates, and a rubric to help assess the contribution level of candidates. Each of the categories comes from its own amalgamation of data streams, which the Instacart trio helped identify, weigh and finetune.
Tailor Market Data
Among the three categorical inputs for compensation at Instacart, market data is where the company continues to conduct most of its analysis. In short, its goal is to understand the delta between market rate and what it’s currently paying. While that may sound straightforward, it takes some filtering of the talent funnel to get right. Here’s how Instacart does it:
Give data to get data. To benchmark compensation at the earliest stages of a company, you may not have a clear idea of who you’re competing with for talent or your own historical candidate data to mine. “There are companies, such as Advanced HR or Radford that run compensation surveys, aggregate the responses and then return it back to the participants. Early on, Instacart found this survey data especially useful,” says Stanley.
“As a Series A or B company, this hits a sweet spot, both in terms of reliability and affordability — between your own guesswork and running your own study. I’d avoid other sources that don’t follow a similar methodology or haven’t run these studies for years because, even if they give a ballpark range, I’ve found that the data isn’t very trustworthy or interpretable,” says Baier.
- Takeaway: If you’re within your early funding rounds and most of your team is working on the product, consider sticking with an offering like Radford Surveys or Option Driver. It’ll provide reliable data and not tax your limited resources. If you have bandwidth or want a more precise formula for compensation, continue on.
Gut-check with your peer group. Most compensation surveys or products allow you to filter by city or zip code to make national data more relevant to where a company is located. “That’s a good start, but region doesn’t equal peer group. Even if you’re able to compare to a set of specific companies in a database, we’ve found it helpful to reach out to HR or People Ops contacts at other companies,” says Baier. “Most are surprised that dialing a counterpart at another company — even a competitor — leads anywhere. But this is not to exchange comp numbers. It’s to level set on what data sources work, methodologies they’ve tried and challenges they face regarding compensation. You’ll learn as much from companies at your stage, as those slightly earlier and later in their growth. We’re all trying to figure this out and stay current with our comp strategy.”
- Takeaway: If you have the time, go beyond fine-tuning filters in your comp survey databases. Pick up the phone and call your peer group to compare processes. Here are some questions that Baier asks herself to get a first cut at Instacart’s peer group: Who are your competitors — for talent, not just business? Who has a similar valuation? Who is using similar data sources to make their decisions?
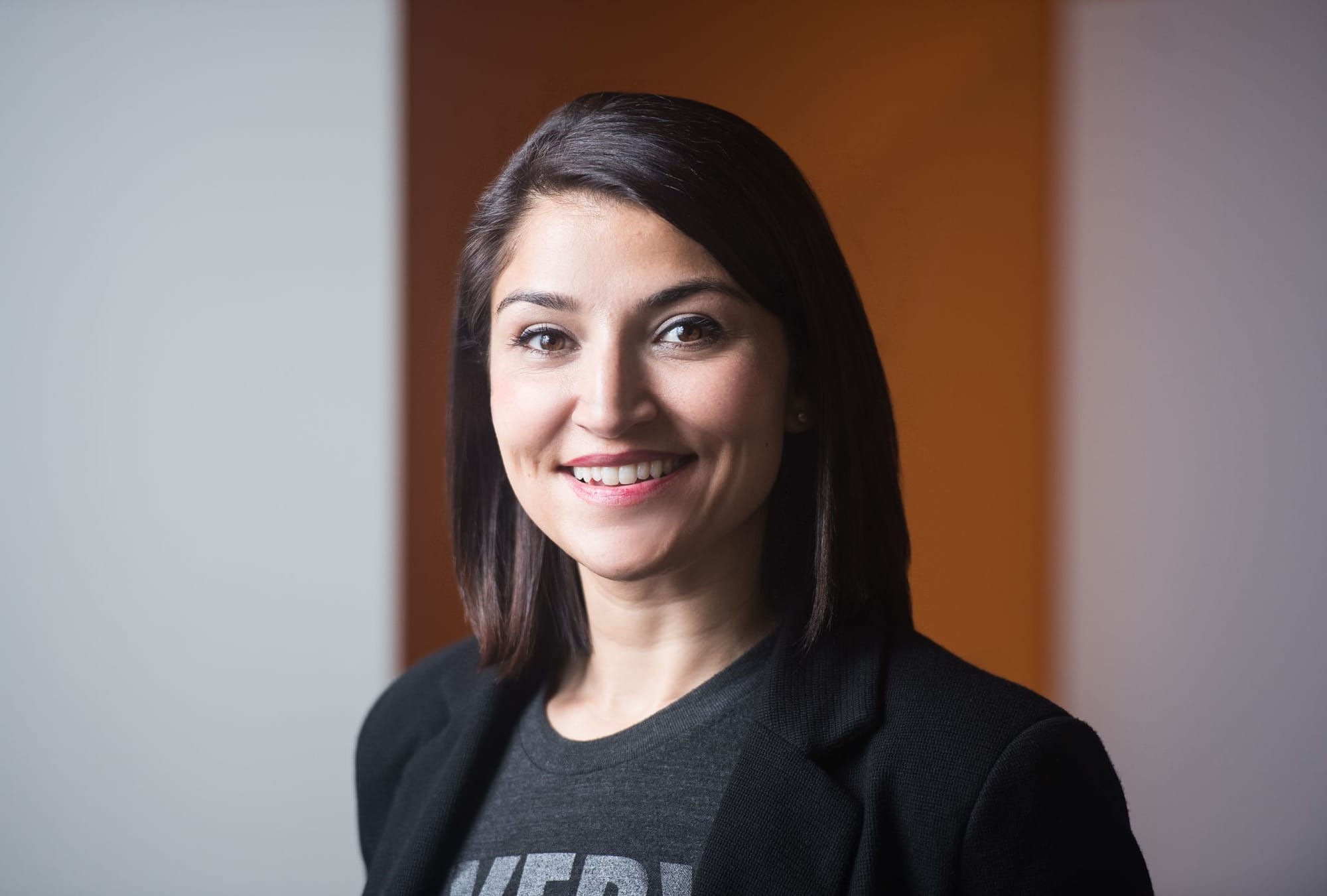
Fire up a regression analysis. This is where Instacart has leveled up its comp strategy. “Initially, what we aimed to do was start from the national data and come up with some factor to apply to get us from national to San Francisco [where Instacart is based], and from there to our peer set of private, growth-stage B2C tech companies. But here’s what we realized: that factor is going to change from one role to another,” says Stanley.
“It’ll vary across seniority, the function, the role — a whole bunch of different attributes,” he says. “So coming up with those factors is essentially the challenge. And we ended up using more data science building regression models that sit on all the data points that we have. Just trying to smooth out this data and impute the data we don't have.”
So here’s what Stanley, Nir and Baier did instead and recommend:
- Start with your own blend of sector data to determine your peer set — and cash versus equity formula. “Early-stage startups may only benchmark with private companies. But at a growth stage, you might use a blend of both private and public company data. That’s what we do. Specifically, we benchmark our cash against other private companies. However, we compete for talent against publicly held companies so we use TDC — essentially total cash incentives and equity — to benchmark against public companies,” says Guissu. “We then back out the cash and pay the balance in equity. We believe in the growing value of our equity and that if the company meets its goals, the value of our comp package would exceed what employees would earn at public companies.”
- Assign attributes to this data. “Then we add information to give context by role,” says Stanley. “From the private and public databases, we collect or extract data for all roles. That gives us a sense of the organizational structure of those positions. We capture and categorize the business unit or group, its function, its seniority level — from VP to individual contributor — and also level of education, from recent grad to PhD.”
- Build a regression model. “We then take all of that data and build a regression model that sits on top of it. It’s weighted by how many companies report to every one of those cells,” says Stanley. “We put in factors that will adjust for the geographic region, seniority level, and position vis a vis organizational structure. We then slowly add what we call ‘interaction effects,’ which allow for one variable to change the effect of another — for example, seniority level impacts compensation differently for different positions. That allows you to see how much more a product manager gets paid as she goes from a level three to four to five to six. And how that slope is different for an engineer or an engineering manager. Or how the slope changes just using San Francisco data.”
- Adjust your position and smooth out the data. “This analysis should help you adjust your overall relative position within your peer group that you’re competing against,” says Stanley. “We do something called regularization in order to prevent from overfitting the data. If you don't do that, then you end up with a lot of wild swings in compensation, and even nonsensical results, like comp going down for a specific role with seniority.”
The takeaway is that a regression analysis will allow you to narrow your benchmark to a better competitive cohort while still providing meaningful data for rare positions and seniority levels — and thus a higher degree of confidence that your comp offering, relative to your peer group, is on target. “Let me drill the point home. Say you’re looking for the comp of a level-five machine learning engineer. Nationwide, there may be hundreds of companies that have those people — maybe even thousands. So the data you get back will be the median salary or median total direct compensation, as well as the 75th and 95th percentiles,” says Stanley. “Issue is that’s not who we compete with for talent — we compete in San Francisco against B2C companies like Airbnb, Stripe, Uber, Google, Facebook and Slack. When you slice the data to that cohort, there may not be enough companies remaining for the vendor to provide you with any information, as they have minimum sample sizes for reporting. By running a regression analysis, you can leverage all of the data, including more common levels, similar roles and other geographies, in order to estimate what your real competitive cohort is paying for that role. That’ll give you a more confident estimate of what your compensation target should be.”
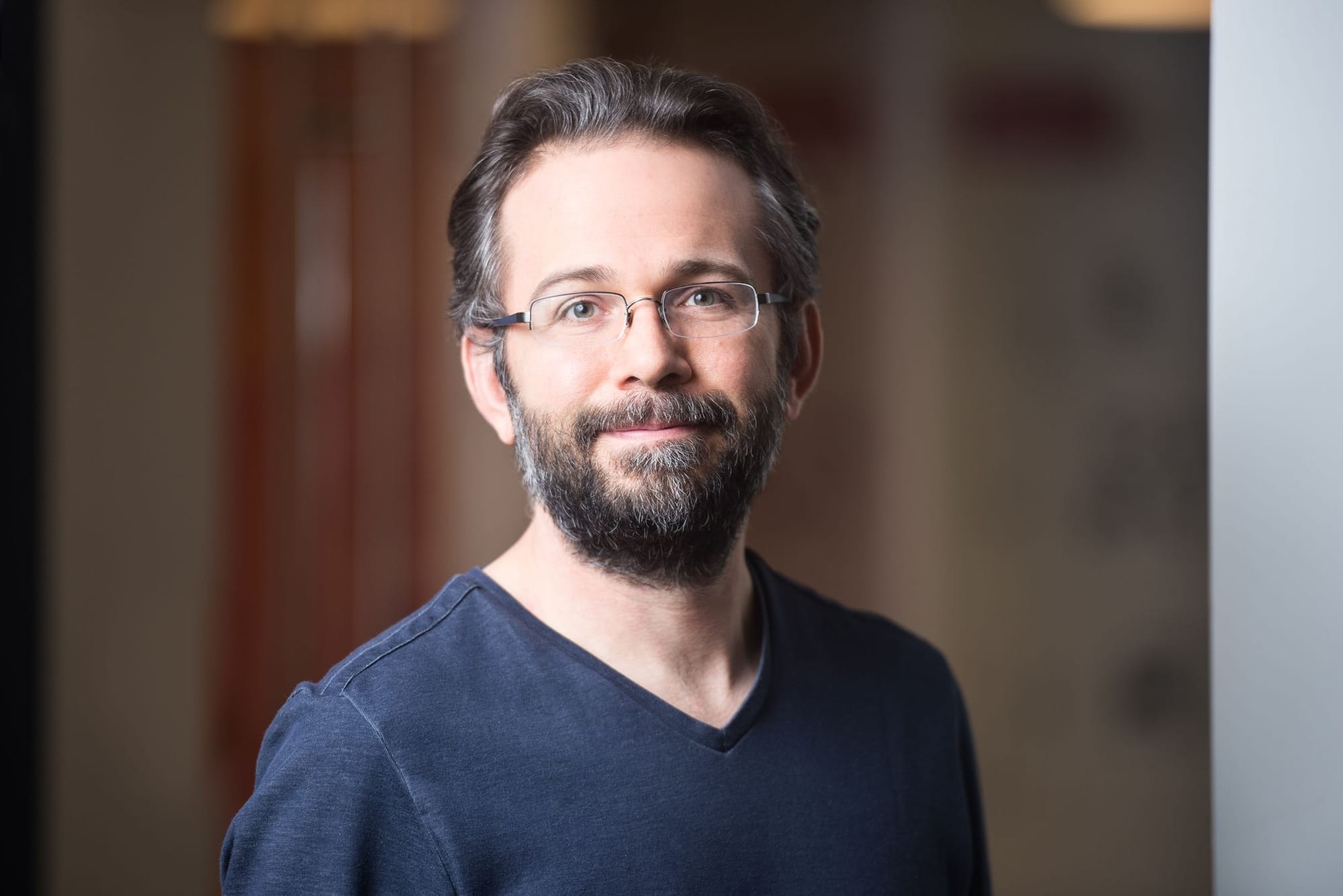
Define Your Market Position and Employee Leveling
Once Instacart backed into its custom competitive cohort, it had more confidence in its methodology — and in its conversations with candidates about compensation. It then had to determine where it wanted to position itself on compensation for any one role, relative to its competitors.
“We've got our custom market data set. But as critical is our explicit process for deciding where we want to be in that market data, which is a different process altogether,” says Nir. “My advice to startups is what we follow ourselves: put yourself in a strong position to win a candidate, but don’t expect to have the best compensation offer every time. Besides, the highest comp doesn’t always win. We’ve seen that by paying fairly, crafting an exciting role and conveying a compelling mission seals the deal. It’s not just about comp. If it is, you may not want that hire.”
Market data on comp isn’t battle-tested by your company. Put it in the ring with candidates for a few rounds. See if it stands.
Establishing the percentile of the market they want to land is an internal decision and a metric that stays confidential to the team at Instacart. However, the other part of the equation is making the levels of their roles — to which compensation is tied — clear to candidates. “Here are a few key principles to consider from the start: first, if you aren't precise about how you are leveling people, no amount of market data is going to help you. You'll just end up comparing apples to oranges,” says Stanley. “Second, once you define precise leveling, compare it — phrase by phrase — to the leveling you can get from third party data. If it doesn’t match, it doesn’t mean you need to change your leveling, but you need to know that it’s different. In some cases, our level 4 was another company’s level 5 — and that has a huge impact on the compensation.”
To date, Instacart has piloted this approach to leveling with its engineering team — and is refining levels and role definitions throughout the rest of the organization. For Instacart Engineering, there are in-depth descriptions for each level, but here they are in a nutshell:
- Level 1: You are just starting your professional career.
- Level 2: You are productive but still learning.
- Level 3: You are a solid, trusted, senior contributor.
- Level 4: You make your team significantly better.
- Level 5: You make your organization (e.g. Engineering) and Instacart better.
- Level 6: You are changing the industry.
Here are a three key principles about these levels:
- Comp is based on contribution, not seniority. “Here’s the question we aim to answer: what kind of impact is this candidate going to have? You’ll note that the descriptions focus on who is impacted by the person’s contributions. For level one and two, the impact is mostly on the individual, and then it expands outward from there,” says NIr. “We use a rubric to gauge how their impact might map to Instacart, not their career to date. It has less to do with their education or years of experience.”
- This is not a linear scale. “In fact, think of it as a logarithmic scale. It’s twice as hard to reach the next level than it was to reach the previous one,” says Nir. “It takes time. Sometimes a long time to move between levels. There’s room to grow within a level. Star performers who exhibit some of the behaviors and skills of the next level usually get promoted within 6-18 months. That’ll be on the longer side for higher levels. Some will top out at a certain level and still be great contributors to the success of Instacart.”
- The principles reinforce the process — for and beyond hiring. “The goals of sharing these levels are to clarify what it means to be more senior at Instacart, help ICs understand how they can grow, support managers as they evaluate their team and establish a practice of fair calibration of comp adjustments,” says Nir. “If you notice, these are not only screens used for the hiring process. We’re just introducing them as early as possible — to candidates before they might join. It’s our first and best shot to introduce ourselves and be consistent.”
In addition to these principles, each level coincides with a certain depth of understanding of company values. “We also attach traits to each level. So, in engineering, we've gotten pretty clear about the different levels that an engineer could be. Though we try to manage to the spirit of these levels rather than the exact wording,” says Jeremy.
In brief, they are ownership (treating Instacart as “your baby”), wisdom (your ability to make good judgment calls), knowledge (chops to help you execute, separate from wisdom), influence (leverage through helping others) and community (role as ambassador and host).
Here’s an example of how this value rubric looks for a level-three engineer:
- Ownership. You are proactive about taking ownership when you see it is needed, and hold others accountable for their ownership as needed.
- Wisdom. You have a solid understanding of your domain, both from a business perspective and a technical perspective. You suggest features and projects that can drive the business forward. You assess the potential impact of proposed features. You understand and can articulate the short-term and long-term technical implications of these features (cost, scale, complexity, etc.). You have sound judgement when making tradeoff decisions.
- Knowledge. You are expected to independently implement solutions, using known techniques/approaches, to pre-defined problems. You have deep knowledge of our technical foundations (e.g. languages, frameworks, etc.). You understand implications of performance, scalability and robustness. You have solid software design and system design skills. You are trusted with selection of tooling, libraries, etc. You know when to write tests and when not to.
- Influence. You are self-sufficient. You have mastered at least one domain/system and are able to provide guidance to others for it. You share your learnings with the team by improving our documentation, capturing tips & tricks, etc. You give great feedback on pull requests. Your personality and attitude positively affect the team.
- Community. You participate in local meetups. You give lightning talks. You help with documentation for open source projects. You present regularly internally at engineering or functional lunches.
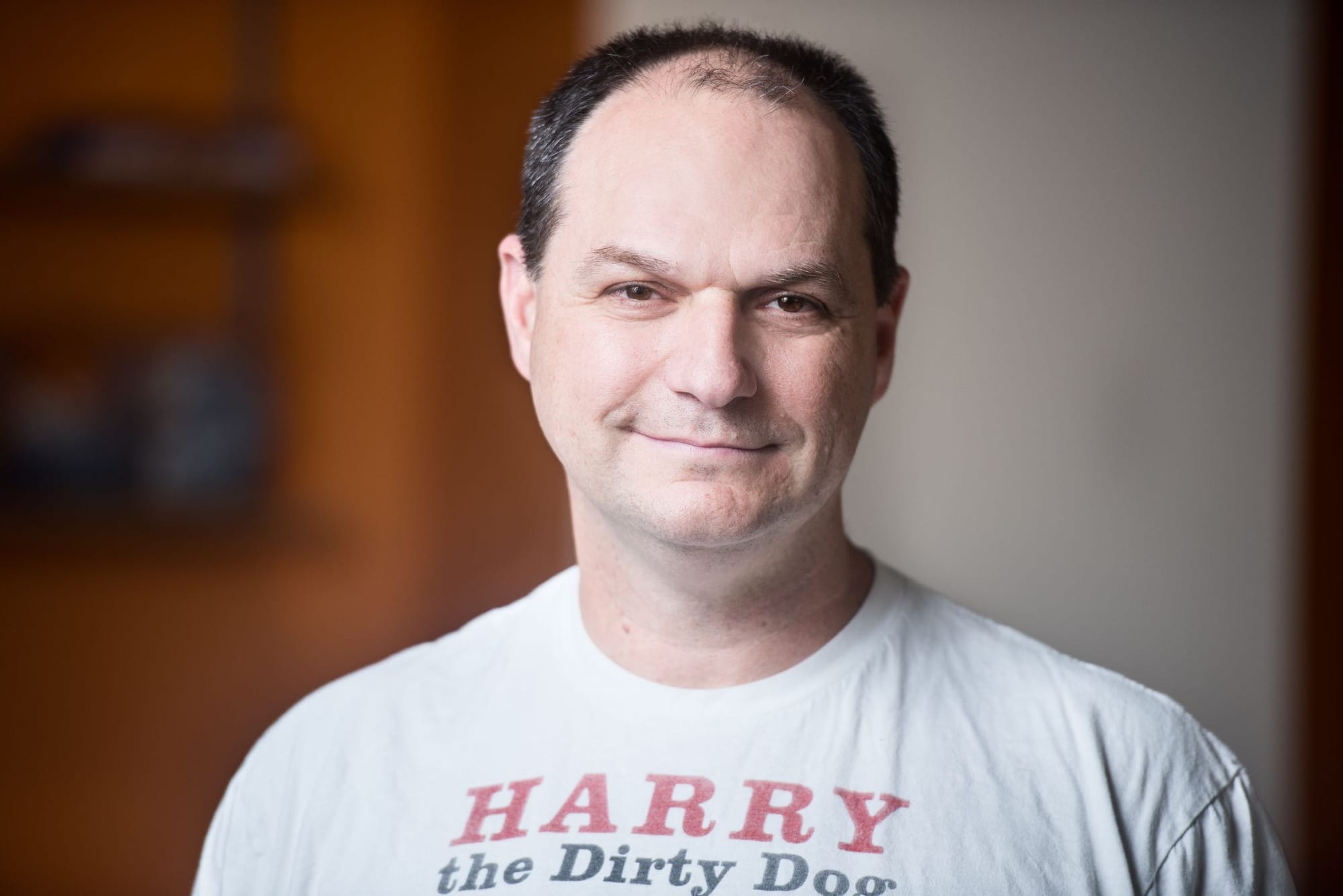
Stress-test Your Comp Strategy
Its algorithm-tweaked market data and values rubric has not only shown that Instacart has been thoughtful about its compensation, but also that it’s designed to be fair. This legwork has been critical in helping the company feel confident to not slide into negotiations with candidates. But even with its firm position on compensation, Instacart still collects insights when candidates do initiate negotiations.
“Market data and candidate negotiations with comp mutually validate each other. Pay close attention to the offers that aren’t immediately accepted, but not declined. If your offer is in play, that means you’re likely in range. Fielding compensation asks from candidates or discussing their other offers will only give you more details about your compensation target and help weatherproof it for future roles,” says Nir. “For example, early on we realized that we were consistently below market for certain analytics roles after conducting a number of interviews. That served as a signal, so we revisited our definition of the role and tweaked our algorithm and custom blend of public and private data to get to compensation target to the correct level.”
One key caveat: don’t collect information to negotiate an individual offer, but to calibrate how you give offers going forward. “We find that candidates generally offer what they currently earn and are looking to make — often during and certainly after the interview process. They want us to know this information and we want them to know we pay fairly. So, we note those expectations not only for that individual conversation, but also as data to improve our overall compensation strategy,” says Stanley. “But here’s what’s important: we say clearly upfront with engineering candidates that we aim to fairly compensate, but don’t negotiate. If they still want to push back, we’ll take note and collect this data, even if they decide not to take the offer. If we find we can't close multiple candidates with the ranges we have, we reevaluate our role definitions, leveling, data and process. But we won’t decide that on the spot in an interview. Our ability to keep internal parity on comp is on the line.”
Even if we want a candidate, we want a consistent compensation standard across our organization more. We don’t want to rock the entire boat for one passenger.
The art of being firm and flexible
A policy against negotiating doesn’t mean that it’s a non-starter if a beloved candidate won’t budge on higher comp expectations. Here are a few ways to approach that situation:
- Give options on the equity/salary balance. “If they are looking for greater compensation, salary is only one lever. What candidates can do is trade cash for equity. We’ve got a formal process that involves a conversation between these options. We speak about it in terms of lifestyle and risk. For example, if you need more cash because you have a mortgage or family responsibilities, you can trade equity for cash. But if you want to take a bigger bet on the company, you can also do that,” says Nir. “About 20% of our engineers choose to alter their equity/salary balance — and of those, 75% choose more cash, for life stage reasons and because our offers are equity-heavy. It’s key to know why people choose to rebalance those parts of their comp. We want to know their reasons, not only to have the data, but so we can better know how to support them.”
- Let the market catch-up with overcompensated incumbents. “If you’re like most startups, you’ll have early employees or one-off hires who may be compensated more than what what is now standard,” says Stanley. “If you’re moving to consistent compensation for each level, let them be outliers in the short term. If you offered an employee too much money, don’t lower their comp. That’s your mistake; don’t make employees pay for it. For the scenarios where we’ve over-compensated people, we will let them stay at that level until the market rises to them. And again, if we under-compensated them, we raise their compensation to market.”
Make Equity More Accessible, Like Cash
Instacart has two practices that it’s found to make a big difference with the equity component of comp. The first is a worksheet that outlines the entire benefits of a position’s compensation. This not only includes salary and equity, but also the company’s contributions to health care, budget for professional development and other perks like meals. A worksheet similar to the following document is shared with candidates:
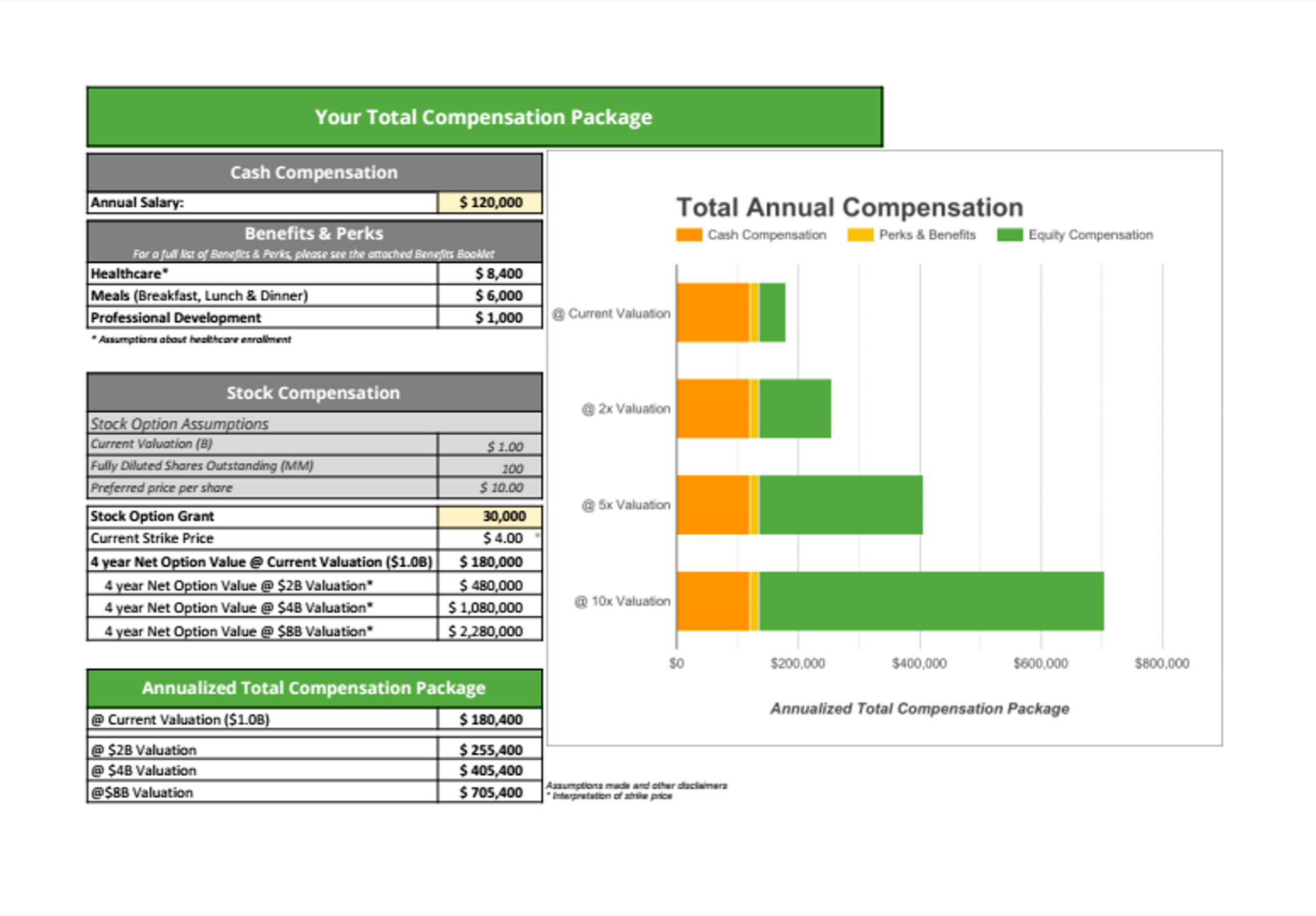
One of the most helpful components of the total compensation package document is how the company has calculated the value of the candidate’s stock options at different valuations. “As employees receive grants post-hire in the form of promotion or refresh grants, we are starting to issue updated worksheets so they can continue to track the value of their equity," says Baier. “In addition, we’re doing a series of stock option workshops, where our executives go through a step-by-step explanation of how stock options work, such as what’s the vest date versus a grant date. It dives into how one calculates valuation. These happen in small groups of about 20 people, so employees can ask questions.”
Second, Instacart has joined a growing number of forward-thinking tech startups, like Coinbase, Pinterest, Asana, Gusto and Amplitude by extending 90-day window provision, during which most departing employees at startups must exercise their vested stock options, to 7 years or more. “A 90-day window typically leads employees to forfeit their stock because they can’t afford to buy it or incentivize them to stick around even though they’re unhappy. The former disempowers the employee. The latter creates a ‘rest and vest’ mentality that diminishes productivity and erodes the brand,” says Baier. “So we made the decision to expand the exercise window to seven years. We want our employee’s equity to be compensation and a retention tool. For both, there’s no need for golden handcuffs. For the equity that’s vested, we want employees to feel like they have flexibility over it and their destiny. Of course, we hope that's at Instacart but if it's not we don't want the reason they’re staying is because they can't afford to leave.”
Track Former Employees Like Alumni
Candidates are hired and employees fulfill their tenure. If they decide to leave, Instacart also uses any departures as opportunities to refine their compensation strategy, so that it can keep its attrition rate within the guardrails of peer companies. “We track attrition. Attrition data is imperfect and horribly lagging, but it’s one more data source to help refine our comp strategy,” says Guissu. “It’s not often that people tell you they leave because they’re getting paid more in another role, but it’s still something we want to track. But mostly, we want to make sure that our attrition rates are not disproportionate to what other companies are experiencing. If it was — and we started to see big gaps in the market data relative to what we’re paying — we’d then be able to put two and two together and adjust. Attrition is another data point to help validate our data-driven model.”
My goal is that people don’t think about comp. They just know they’re being treated fairly and can confidently allocate their mental energy to growing the business.
Adding it All Up
To the startup leader ready to hire: before you’re under the gun to fill key roles and tempted to inch outside a role’s comp band for that must-have engineer, do some legwork. A transparent, trustworthy compensation system takes effort, but pay dividends. Comp data from third-party providers is an effective place to start. Use a blend of private and public data to create a more custom peer group against which to benchmark. This cohort should reflect your stage of growth and the types of companies you compete with for talent. Layer on attributes to the roles within these cohort of companies, such as region, seniority and function. Build a regression model — and add interaction effects to better manipulate variables and regularization to keep from overfitting the data. Map this intelligence to your employee leveling by function. Then stress-test these compensation targets with your candidates and employees. That means tracking metrics such as salary expectations, competing offers and attrition rates. All of these inputs help ground your algorithm in reality and more effectively — and equitably — compete for talent.
“To invest in a comp methodology is to is to invest in your people — the ones you’ve hired and are yet to hire. But I hear of early-stage startups jumping straight into hiring without giving serious thought to a holistic comp philosophy. That’s a quick way to mortgage your future hires for your early ones, by unintentionally creating ‘incumbents’ who’ll be comp outliers down the line. That may not hurt immediately, but you’ll feel the pain compound over time,” says Stanley. “Craft a more customized, data-driven compensation methodology as soon as you can. You’ll create a more durable system, built from confidence that you’re paying fairly and a consistency that both candidates and employees recognize. If you’re doing it right, you’ll not only determine how employees are compensated, but start to touch on how they feel valued. That’s when your currency is not only the compensation you offer, but the culture you’ve built. That’s powerful.”
Photography by Bonnie Rae Mills.